AI image evaluation vs. manual microstructure analysis - advantages and disadvantages
Discover how artificial intelligence is transforming metallography: An in-depth comparison between traditional manual image analysis and modern AI-powered methods in materials science. Learn more about the pros and cons of both approaches in our latest blog post.
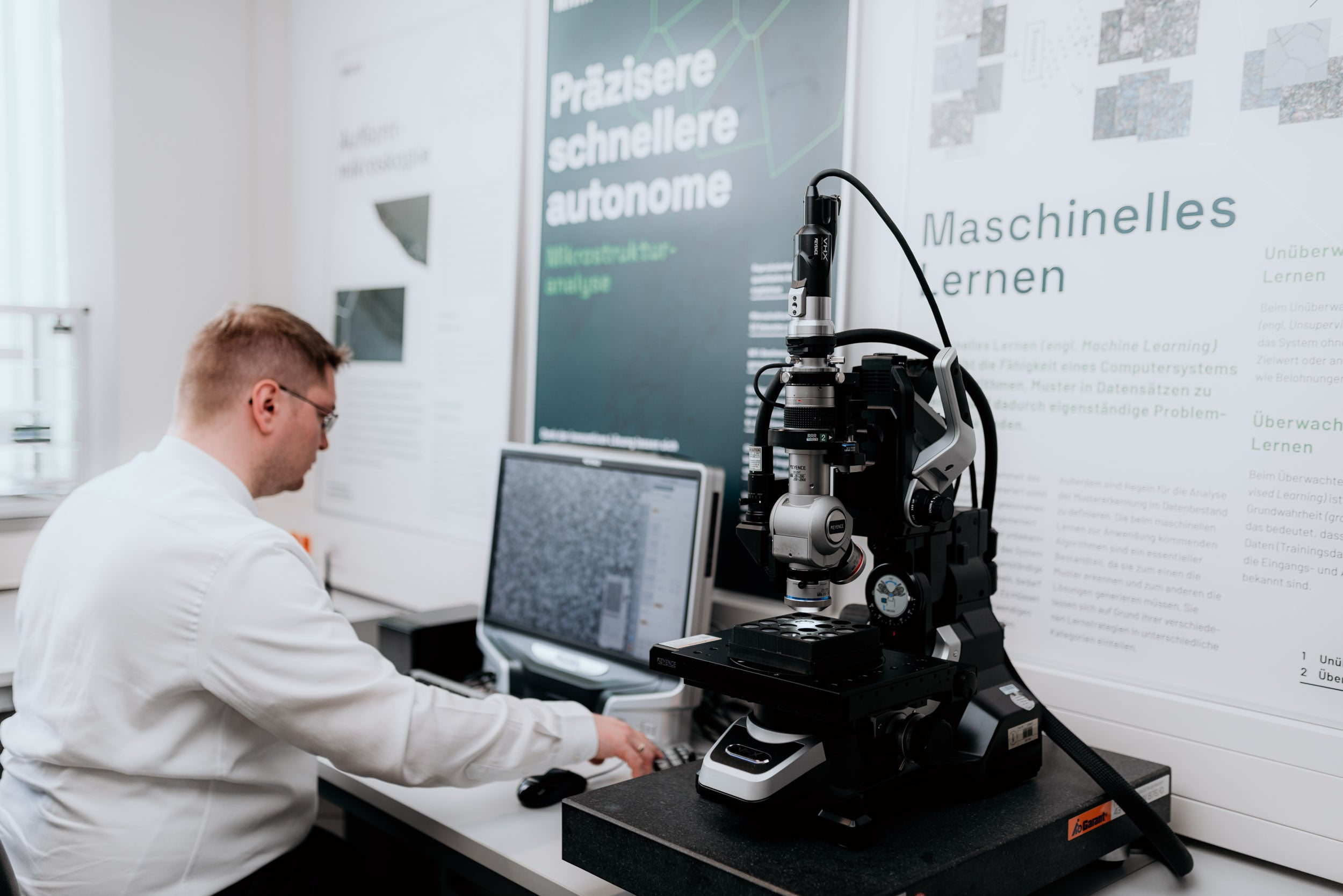
Navigation
With the increasing integration of technology and artificial intelligence (AI) into our everyday lives, the question often arises as to how these developments compare to traditional methods. One particularly interesting field is image analysis, where AI systems are increasingly being used.
Die Metallografie ist ein entscheidender Bereich in der Materialwissenschaft und hat sich durch den Einsatz von Künstlicher Intelligenz (KI) signifikant weiterentwickelt. Traditionell basierte die Analyse von Mikrostrukturen auf manuellen Techniken, die von erfahrenen Fachleuten durchgeführt wurden. Mit dem Aufkommen der KI-Technologie in der Bildanalyse stellt sich nun die Frage: Wie verändert die KI-Auswertung die Metallografie im Vergleich zur manuellen Auswertung von Mikroskopbildern? In diesem Blogbeitrag beleuchten wir die Vor- und Nachteile beider Methoden.
AI image analysis
Benefits
Time saving - boosting productivity in metallography:
KI-Systeme können große Mengen von Bildmaterial in kürzester Zeit verarbeiten, was bei manueller Analyse unmöglich wäre. Dieser Vorteil kann sich auch in der Metallografie zu Nutze gemacht werden, wo eine schnelle und effiziente Auswertung von Mikrostrukturen entscheidend ist. Solche Systeme können dabei helfen, große Mengen an Bilddaten in kürzester Zeit zu verarbeiten, was für die Qualitätskontrolle und Materialforschung von großer Bedeutung ist.
Consistency - Making sure image analysis quality stays consistent
Unlike humans, who become tired or whose attention wanes, the performance of an AI remains constant. This results in a consistent quality of image analysis, regardless of the time of day or the number of images already analysed.
Recognising complex patterns - uncovering hidden details in images
Modern AI algorithms can recognise patterns and details that are difficult for the human eye to identify. These advanced systems are specially trained to identify subtle patterns, fine structures and even minor deviations in images that often go beyond the ability of the human eye to recognise.
Lernfähigkeit – Steigerung der Analysegenauigkeit über die Zeit
Modern AI systems, especially those based on machine learning, can learn from each analysed image and improve their accuracy over time. This enables them to recognise even complex patterns that are difficult for the human eye to identify.
Automated documentation - support for consistent archiving processes
The results are automatically documented, which enables sustainable data archiving. It is ensured that all relevant information is archived systematically and consistently. This is particularly important in areas where the traceability and long-term availability of data is crucial or is used for the continuous improvement of products and processes.
Downsides:
Cost-benefit analysis - evaluation of the economic efficiency of AI systems
The development and implementation of AI systems for image analysis can be expensive, especially in terms of the hardware and software required and the expertise needed to train the systems.
Vorteilhaft kann daher die Nutzung fertig trainierter Analysemodule sein. Diese werden beispielsweise von MiViA angeboten.
Data quality and bias risks - ensuring reliable AI results:
KI-Modelle, insbesondere im Bereich des maschinellen Lernens, lernen aus den Daten, mit denen sie trainiert werden. Wenn diese Trainingsdaten unvollständig, veraltet oder voreingenommen sind, kann das Modell irreführende, ungenaue oder verzerrte Ergebnisse liefern. Beispielsweise kann ein Bilderkennungssystem, das nur mit Bildern bestimmter Werkstoffe trainiert wurde, Schwierigkeiten haben, Mikrostrukturen anderer Werkstoffe zu erkennen.
Fehlende menschliche Intuition – Grenzen der KI in komplexen Analysekontexten
AI systems are usually very good at recognising and classifying patterns in data, but they lack human intuition and understanding. In situations where context or common knowledge plays a role, AI systems can struggle to make appropriate decisions.
Limitations of traceability - the black box problem
Many advanced AI models, especially deep neural networks, are very complex in their operation and difficult for humans to interpret. This leads to the so-called black box problem, where it is difficult to understand how and why the AI system has arrived at a certain result. This can affect trust in AI decisions.
Susceptibility to outliers - challenges with atypical data in AI systems
AI systems can react sensitively to data that deviates significantly from their training data. Such outliers can lead to unexpected or erroneous results. The challenge is therefore to develop AI models that are robust enough to deal with the inherent variability and rare but significant features in microstructure data without losing accuracy
Manual image analysis
Benefits
Flexibility and adaptability
Humans have the unique ability to adapt quickly to new and unexpected situations. Unlike AI systems, which are based on their training data, human analysts can interpret images in context and react to unforeseen changes or anomalies. This flexibility is particularly important in areas where the data is variable or the analysis conditions change frequently.
Experience and contextual understanding - key components of manual analyses
Human experts bring their experience and deep understanding of the framework in which the images are analysed. They can recognise subtle nuances and understand the meaning of image content in a broader context. This ability enables them to gain unexpected insights and critically evaluate the results, which is particularly important in complex or specialised applications and scientific research.
Independence from training data - direct analysis capability without preconditions
Another advantage of manual image analysis is that it is not dependent on extensive data sets for training. While AI systems require extensive and often specialised training data to function effectively, human analysts can interpret images directly without such preconditions. This makes manual analysis more flexible and applicable in situations where extensive historical data is not available or where the data is too unique to be effectively analysed by an AI. Translated with www.DeepL.com/Translator (free version)
Downsides:
Time expenditure and efficiency limits - challenges of manual analysis
Manual image analysis is time-consuming and can be impractical with large amounts of data. In addition, human attention can wane during lengthy analysis processes, which can lead to errors. Fatigue and monotony can affect the accuracy and reliability of the analysis, especially for repetitive or detail-intensive tasks.
Subjectivity and inconsistency - weaknesses of human analyses
The interpretation of images by humans is inherently subjective. Different analysts may interpret the same images differently, which can lead to inconsistent results. The quality of manual image analysis can vary depending on factors such as the analyst's experience, their current state and the specific situation.
Scaling problems - limited capacities in manual data processing
A human can only process a limited amount of image material in a realistic amount of time. In contrast to automated systems, such as AI-based analyses, manual image analysis is difficult to scale. Analysing large amounts of data requires a proportional increase in personnel and resources, which can be both logistically and financially challenging.
Susceptibility to errors: risks of human error in the analysis
Despite the undeniable advantages that human expertise and intuition bring, the susceptibility to error remains a critical issue. Metallographers are not immune to errors, which can be caused by a variety of factors such as fatigue, distraction or subjective bias. These errors can manifest themselves in the form of overlooking important details, misinterpretation of data or inconsistent results from repeated analyses.
In areas where precision is paramount, such errors can have far-reaching consequences. It is therefore crucial to recognise the limits of human analytical capability and develop strategies to minimise the risks of human error, whether through complementary AI support or improved training and review processes.
Conclusion
Both AI image evaluation and manual analysis have their specific strengths and weaknesses. In many cases, a combination of both methods can be used to maximise the advantages and minimise the disadvantages. While AI systems can improve the efficiency and speed of image analysis, human intuition and flexibility remain irreplaceable, especially in complex or unforeseen situations. The future of image analysis could lie in a synergistic collaboration between humans and machines, with each method bringing its unique strengths to the process.
We would be happy to arrange a consultation with you to find out whether an AI image analysis is suitable for your needs.