Will AI replace the metallographer? A look at the future of microstructure analysis
Metallography provides important information about the properties, performance and service life of metallic materials. But how does the rapid development of artificial intelligence (AI) influence this traditional material testing? Can AI replace metallographers or will it merely support them? In this blog article, we take a comprehensive look at these questions.
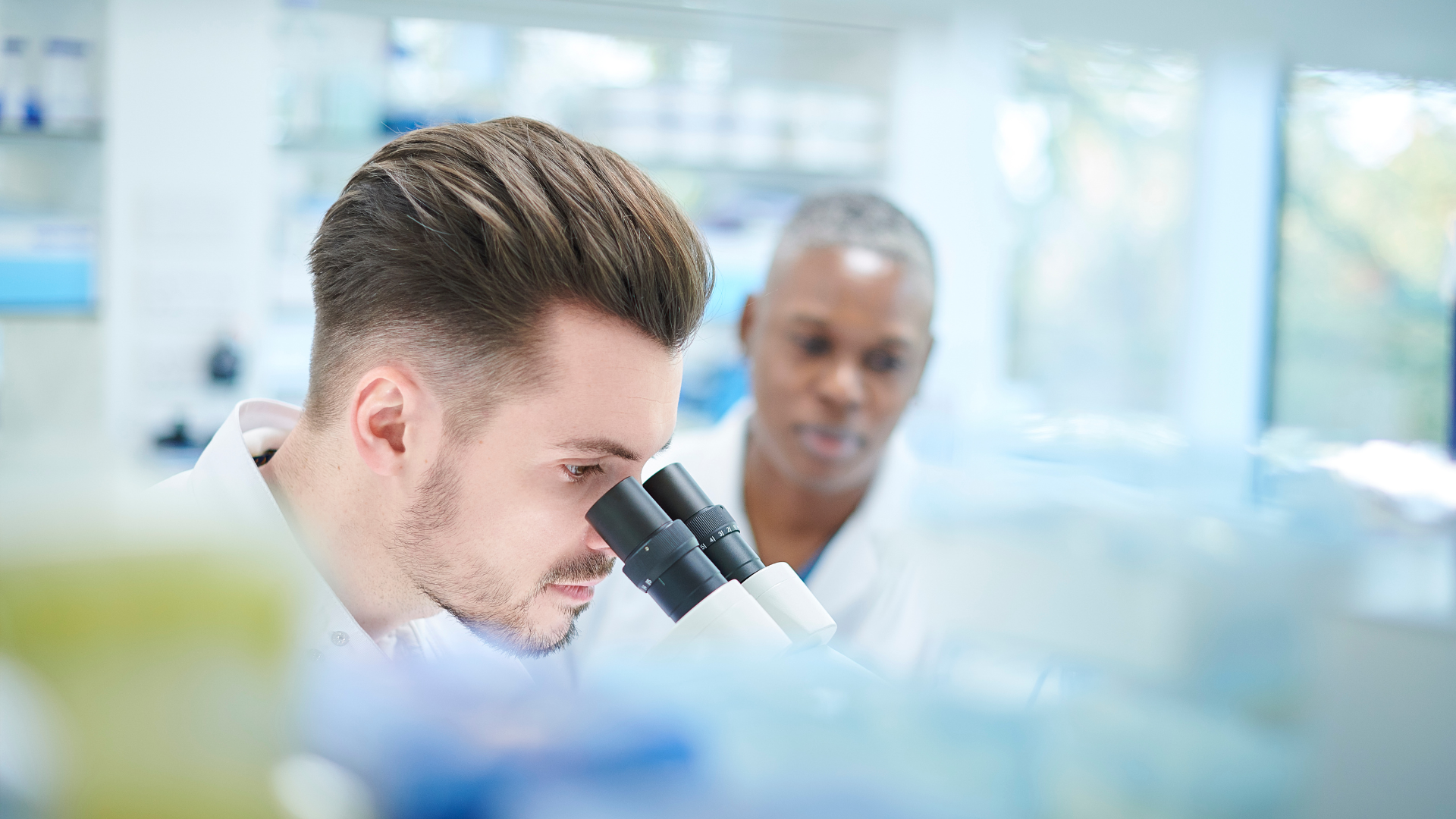
Navigation
The role of the metallographer
Metallographers analyse the microstructures of metals using microscopes and other analytical methods such as scanning electron microscopy (SEM) and energy dispersive X-ray spectroscopy (EDX). These analyses are crucial for quality control, defect analysis and the development of new alloys. By interpreting the microstructures, metallographers can draw conclusions about mechanical properties such as hardness, strength and toughness.
Advancements in Artificial Intelligence
AI has made considerable progress in recent years, particularly in the areas of image processing and pattern recognition. Machine learning algorithms, especially deep neural networks, are able to recognise and analyse complex patterns in large amounts of data. These capabilities predestine AI for use in metallography, where large volumes of image data need to be analysed.
Applications of AI in metallography
- Automated image analysis: AI can be used to automatically analyse metallographic images and classify microstructures. Algorithms can recognise grain and phase boundaries, identify porosities and inclusions and perform quantitative analyses such as grain size distribution.
- Error detection: AI systems can be trained to recognise specific defects in metal samples, such as cracks, cavities or unwanted phases. This enables faster and more accurate defect analysis compared to manual methods.
- Prognostic material properties: By analysing microstructure images, AI models can also make predictions about the mechanical properties of a material in the future. This could significantly accelerate the development of new alloys by enabling researchers and engineers to optimise specific properties.
Benefits of integrating AI
- Increased efficiency: Automated analysis using AI can drastically reduce the time needed to analyse metal samples. This is particularly beneficial in industrial production, where fast results are crucial.
- Objectivity: AI algorithms eliminate human error and subjectivity, leading to more consistent and reproducible results.
- Data processing: AI can quickly analyse huge amounts of data and recognise patterns that would be difficult for a human metallographer to detect.
Challenges and Limitations
Despite the promising applications, there are also challenges when it comes to integrating AI into metallography:
- Data qualityThe accuracy of AI analyses depends heavily on the quality of the training data. Poor or insufficient data can lead to incorrect results.
- Black Box: Many AI models, especially deep neural networks, act as "black boxes", which means that the decision-making process is not always transparent. This can be problematic when critical decisions have to be made.
- Human expertise: Although AI can automate many tasks, human expertise remains essential to validate the results and make complex decisions.
The Future
The future of metallography will probably be a close collaboration between man and machine. AI will not replace metallographers, but will complement and support their work. By combining AI-driven analyses and human expertise, more accurate and efficient results can be achieved.
Here are some future perspectives on how the collaboration between AI and human metallographers could develop:
1. Hybrid working models
Future work in metallography will increasingly involve hybrid models in which human experts and AI systems work hand in hand. Here, AI takes on repetitive and data-intensive tasks, while metallographers carry out complex analyses and interpretations.
- Tasks of AI: Automated data acquisition, pre-analysis and pattern recognition.
- Tasks of the metallographer: Validating the AI results, carrying out complex analyses and deciding on necessary measures.
2. Progress in AI-supported research
KI wird die Forschung in der Metallografie revolutionieren, indem sie neue Wege zur Entdeckung und Entwicklung von Materialien eröffnet. KI kann dabei helfen, Daten aus verschiedenen Quellen zu integrieren und neue Korrelationen und Muster zu entdecken, die mit herkömmlichen Methoden nicht sichtbar wären.
- Material discovery: Using AI to predict new alloys with specific properties.
- Optimisation of existing materials: Application of AI to improve existing materials and optimise their performance.
3. Improved error analysis and quality control
The integration of AI will significantly increase the accuracy and efficiency of defect analysis and quality control. Through the continuous improvement of AI algorithms, defects can be recognised faster and more precisely.
- Real-time monitoring: Implementation of AI-supported systems for real-time monitoring of production processes.
- Preventive maintenance: Prediction of possible material faults and preventive measures to avoid failures.
4. Training and further education
The training of future metallographers will change in order to meet the requirements of AI integration. Metallographers must be familiar with the basics of AI and its application in materials science.
- Training programmes: Development of training programmes and courses covering both traditional metallography and modern AI techniques.
- Interdisziplinäre Ansätze: Förderung interdisziplinärer Zusammenarbeit zwischen Materialwissenschaftlern, Informatikern und Datenwissenschaftlern.
5. Advanced databases and knowledge systems
With the increasing use of AI, extensive databases and knowledge systems are being created that are continuously fed with new data. These systems can serve as a central source of knowledge and facilitate access to historical and current data.
- Centralised databases: Creation of global databases that can be used by scientists and engineers.
- Knowledge management: Use of AI to manage and analyse large amounts of data and generate insights.
6. Ethical and social aspects
The integration of AI into metallography also raises ethical and social issues that need to be considered. These include questions of data security, responsibility and the impact on employment.
- Data protection: Ensuring the confidentiality and security of sensitive data.
- Responsibility: Clear definition of responsibilities when using AI-supported systems.
- Arbeitsmarkt: Anpassung der Arbeitsmarktstrategien zur Unterstützung von Beschäftigten im Übergang zu neuen Arbeitsmodellen.
Conclusion
Artificial intelligence has the potential to revolutionise metallography by improving the efficiency and accuracy of analyses. However, it will not be able to completely replace metallographers. Rather, a symbiotic relationship will emerge in which the strengths of both sides are utilised to overcome the challenges of materials science. The integration of AI into metallography is an exciting step into the future that offers great opportunities, but must also be approached carefully and responsibly.