Intelligent material analysis - insights into the implementation of MiViA for the determination of retained austenite
The implementation of artificial intelligence (AI) in microstructure analysis is a crucial step towards improving the efficiency and accuracy of quality control. This case study highlights the introduction of an AI-supported system for the determination of retained austenite in the production of transmission gears.
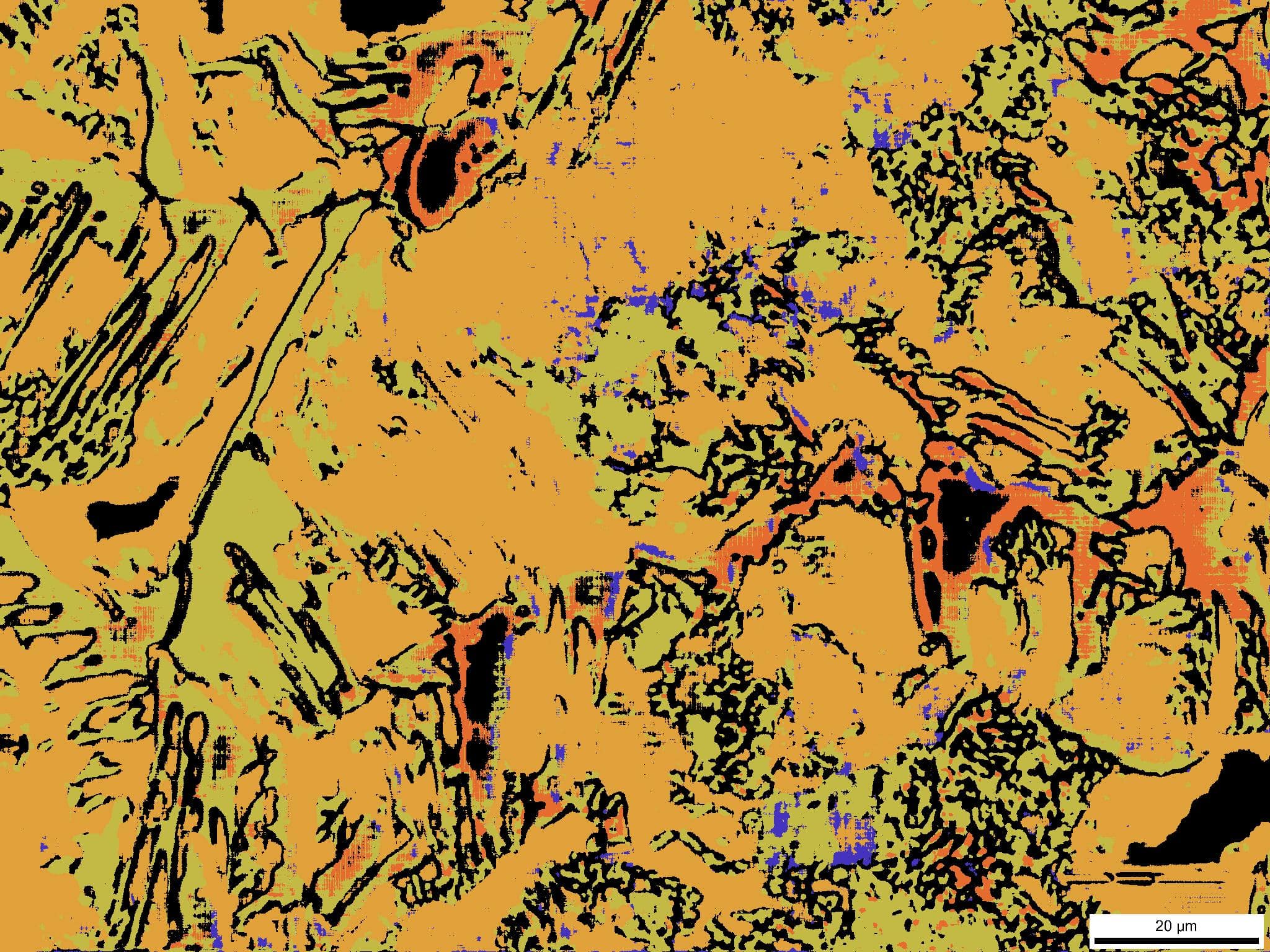
Navigation
Background: The company in question was faced with the challenge of improving the quality of the manufactured gears and simultaneously increasing the efficiency of the processes. Traditional methods of microstructure analysis were time-consuming and required extensive expert knowledge, which led to bottlenecks in production and quality assurance.
Goal: The goal was to implement an AI-supported system to automate and optimise microstructure analysis. The aim was to shorten throughput times, reduce costs and improve the accuracy of quality control.
Basics of retained austenite
Significance, determination and effects in materials science
1 What is retained austenite? Retained austenite is a specific phase in steel and cast iron that remains after heat treatment, especially after quenching. This metastable phase, which consists of a face-centred cubic lattice, can transform into other phases such as martensite under certain conditions.
2 Why is retained austenite determined? Determining the retained austenite content is critical to understanding and controlling the mechanical properties and stability of the material. Excessive retained austenite content can lead to undesirable properties such as lower hardness, reduced wear resistance and potential dimensional changes in service. Conversion to martensite due to mechanical stress or temperature changes can lead to volume changes that cause internal stresses and microcracks. This can affect the service life and performance of the material.
4. How is retained austenite measured? Retained austenite is usually determined using X-ray diffraction. Alternatively, magnetic measurement methods or hardness measurements can also be used for approximate determination. Another important method is the evaluation of light microscopic images. In this technique, microstructure images of the material are taken under a light microscope and analysed. By observing specific patterns and structures in the microstructure, conclusions can be drawn about the proportion and distribution of retained austenite.
Application
Improving the quality of manufactured transmission gears while simultaneously increasing the efficiency of quality control
Initial situation: Gearwheels are manufactured from steel. It is crucial for their function that very precise dimensions are maintained, as they must fit exactly into the transmission systems.
Heat treatment process: The gears undergo heat treatment to achieve the desired mechanical properties, such as hardness and wear resistance. This process typically involves heating the steel to a high temperature to achieve an austenitic phase, followed by rapid quenching to form martensite.
Entstehung von Restaustenit: Während des Abschreckens kann es vorkommen, dass nicht der gesamte Austenit in Martensit umgewandelt wird. Ein Teil des Austenits bleibt als Restaustenit im Gefüge des Zahnrads zurück. Dieser Restaustenit ist bei Raumtemperatur metastabil.
Maßänderungen durch Umwandlung: Im Laufe der Zeit oder unter mechanischer Belastung kann sich der Restaustenit in Martensit umwandeln. Da Martensit ein größeres Volumen als Austenit hat, führt diese Umwandlung zu einer Ausdehnung des Materials an den Stellen, wo die Umwandlung stattfindet. Bei einem Präzisionsteil wie einem Getriebezahnrad kann diese geringfügige Volumenänderung ausreichen, um die Maße des Zahnrads zu verändern und es außerhalb der erforderlichen Toleranzen zu bringen.
Folgen: The gear wheel may no longer fit exactly into the gearbox, which can lead to increased wear, noise or even failure of the gearbox.
Solution approaches: Precise control of the heat treatment process and subsequent verification of the retained austenite content are crucial in order to avoid such dimensional changes.
Intelligent material analysis
Implementation of MiViA for the determination of retained austenite
Preparation: Team composition and goal definition
Putting together an interdisciplinary team of process engineers, IT experts and quality control staff. Together, the team defined the specific objectives of intelligent material analysis, such as improving objectivity and increasing efficiency in the quality control process.
Data collection: Collection, Categorization, Quality control
Collecting microstructure images and associated process parameters from past and current heat treatment processes. The data was classified according to various criteria, such as material type, treatment type and properties achieved, in order to build a structured data set representing the most common analyses from everyday metallography. The data was then checked for completeness and accuracy to ensure high quality validation data.
Testing the AI: test analyses and fine-tuning
Carrying out test runs with the previously defined data set to assess the accuracy and reliability of the AI analysis. Based on the results of the test runs, some minor adjustments were made to the preparation and recording process, which led to a further improvement in the analysis results.
Implementation and integration: System integration, training and documentation
Integration of the AI system into the existing quality control process. The AI analysis was integrated into the existing quality control infrastructure, taking care to ensure seamless integration into the workflows. Training sessions were then organised for the metallography staff to ensure that everyone involved was familiar with the operation and possibilities of the new technology. A short user manual was also created to support the staff.
Monitoring and optimisation: performance monitoring, feedback collection and optimisation measures
The quality of the results is regularly checked to ensure the effectiveness and accuracy of the AI analysis and the findings from the continuous determination of retained austenite have been used to optimise heat treatment processes and the quality control procedure.
Results
What could be achieved by implementing intelligent material analysis?
Improved accuracy and consistency:
As the AI algorithms can process and analyse large amounts of data quickly, more precise and consistent results could be generated when determining the retained austenite content.
Optization of the heat treating processes:
With AI analysis, it was possible to analyse numerous samples in a very short time, which helped to identify the optimal conditions for heat treatment.
Automated quality control:
By automating the process, the efficiency and objectivity of the quality control could be improved. At the same time, cost per analysis could be reduced.
Reduction of rejects and rework:
The comprehensive analyses of the retained austenite content led to a significant improvement in the accuracy of quality control, resulting in a reduction in scrap.
Integration with other manufacturing processes:
The AI analysis could be easily integrated with other digital systems in the production environment, eliminating further sources of error.
Data-driven decision-making:
The data and analyses provided could be used for strategic decisions on process improvement and the development of new products.
Fazit: Die Implementierung von MiViA zur Bestimmung von Restaustenit bei der Herstellung von Getriebezahnrädern war ein großer Erfolg. Sie führte nicht nur zu einer Steigerung der Effizienz und Genauigkeit in der Qualitätskontrolle, sondern ermöglichte auch eine tiefere Einsicht über das Werkstoffverhalten. Diese Case Study zeigt, dass die Integration von KI in traditionelle Industrieprozesse erhebliche Vorteile mit sich bringt und ein Schlüsselfaktor für zukünftige Innovationen in der Prozess- und Materialentwicklung ist.