AI in the steel industry: a new era of innovation and efficiency
In the steel industry, AI solutions are already being used in various areas to improve efficiency, productivity and sustainability. Here are some examples of AI applications that are being used in steel production:
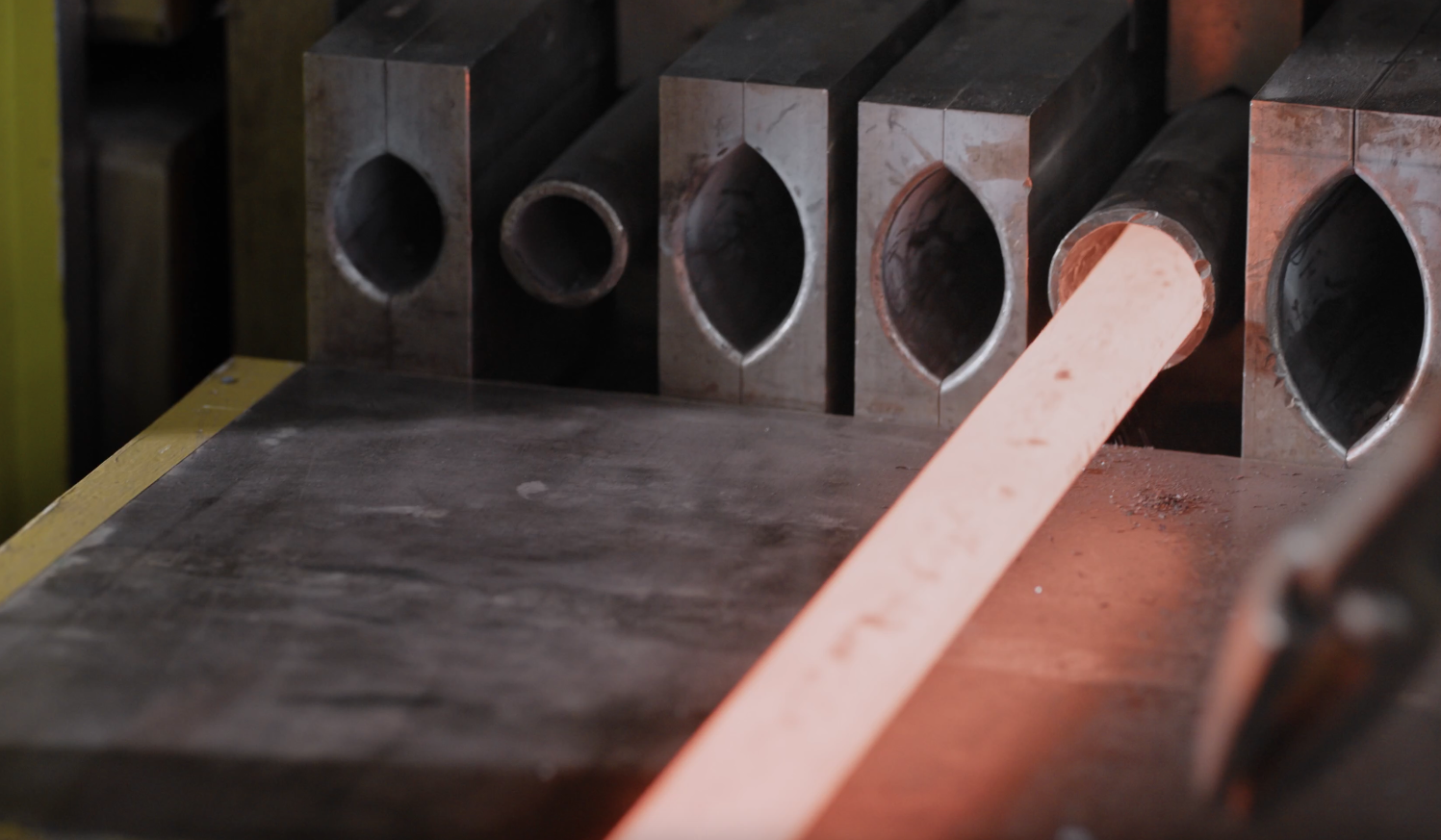
Navigation
Table: AI applications in the steel industry
Areas | Application | Benefits |
Process optimization | Real-time analysis of energy consumption and optimisation of operating parameters | Reduction in energy consumption and production costs |
Quality assurance | Image recognition algorithms for error detection | Early detection and correction of defects, ensuring product quality |
Maintenance and servicing | Predicting maintenance requirements by analysing sensor data | Avoidance of unplanned downtimes, extension of the service life of system components |
Supply chain and demand forecast | Improving demand forecasts and optimising logistics | Reduction of storage costs, optimisation of production planning |
Sustainability and environmental protection | Optimisation of processes to reduce energy consumption and emissions | Contribution to environmental protection, reduction of the CO2 footprint |
Process optimization with AI
Process optimisation is a key area of application for AI in the steel industry. AI models play a crucial role in the analysis and optimisation of energy consumption in real time. These models are able to learn from a wealth of data and recognise patterns. By continuously monitoring operating conditions, these intelligent systems can suggest precise adjustments to operating parameters in order to minimise energy requirements. This is particularly relevant in a sector such as steel production, where energy consumption plays a critical role from both an economic and environmental perspective.
- In addition to energy efficiency, AI-supported systems also make a significant contribution to improving production control. By predicting process variables, AI systems can optimise the operation of blast furnaces and rolling mills.
These predictions are based on complex algorithms that take into account historical production data, current operating conditions and even external factors such as market demand and raw material prices. The ability to predict future conditions and needs allows operators to proactively make adjustments that not only save energy but also increase product quality.
Quality assurance through advanced technologies
The quality of steel products is significantly improved through the use of AI technologies. By implementing AI in quality assurance, steel manufacturers can not only increase the efficiency of their production processes, but also ensure higher product quality. The ability of AI to analyse complex data, make precise predictions and make adjustments in real time is transforming traditional approaches to quality assurance and setting new standards in the steel industry.
- AI-supported image recognition algorithms analyse images within seconds, enabling much faster and more accurate defect detection compared to traditional manual inspection methods.
In addition, AI provides constant, relentless monitoring that improves the consistency and reliability of quality control, unlike human inspectors who can be subject to fatigue or subjective judgement.
Servicing and maintenance: prevention instead of reaction
By analysing sensor data, AI systems can predict the optimal time for maintenance work, which avoids unplanned downtime and extends the service life of critical plant components. These accurate predictions make it possible to anticipate potential failures and take preventive action before unplanned downtime occurs. The use of AI in preventive maintenance not only helps to extend the service life of critical plant components, but also optimises the overall efficiency of production plants.
- By minimising downtime and reducing repair costs, preventive maintenance through AI supports more sustainable and cost-efficient operations, which ultimately strengthens the competitiveness of companies in the highly competitive steel industry.
Optimisation of the supply chain and demand forecasting
AI models not only improve the prediction of market demand, but also optimise routes and warehousing in logistics. These models are able to recognise complex patterns and trends in large amounts of data derived from historical sales data, market analyses and even socio-economic indicators. The resulting accuracy in demand forecasting enables steel manufacturers to precisely align their production plans with future market demand, avoid overproduction and thus minimise storage costs. In addition, AI-supported optimisations in logistics help to refine transport routes and warehousing strategies.
- By analysing traffic flows, delivery times and storage capacities, AI systems can identify and implement the most efficient logistics paths, leading to a further reduction in operating costs.
These improvements in supply chain optimisation and demand forecasting through AI not only enable steel manufacturers to use their resources more efficiently, but also to respond more flexibly to market changes and significantly reduce production costs. In an industry where margins are often under pressure, such technological advances offer a significant competitive advantage and contribute to the long-term sustainability and success of companies.
Sustainability and environmental protection
Another significant advantage of AI is the promotion of sustainability and environmental protection. More efficient production processes and optimised firing methods can significantly reduce energy consumption and CO2 emissions. Automated systems also monitor compliance with environmental standards and thus contribute to responsible resource management.
Challenges and solutions
Despite the promising benefits, steel manufacturers face challenges when implementing AI. These include high initial investments, a shortage of skilled labour and concerns about data security. To overcome these challenges, close collaboration between the steel industry, AI developers and educational institutions is required. Investment in education and training is crucial to build a skilled workforce that can handle the new technologies.
Herausforderung | Lösungsansatz |
High initial investments | Partnerships with technology providers and utilisation of subsidies |
Lack of skilled workers | Investment in staff training and retraining; cooperation with universities |
Data protection and security | Implementation of advanced security protocols and regular reviews |
Integration in existing systems | Development of modular and scalable solutions; use of middleware |
Change resistance within the company | Change management strategies; transparent communication of benefits and objectives |
Sustainability requirements | Use of AI to optimise energy consumption and promote the circular economy |
Conclusion
The integration of AI into the steel industry marks the beginning of a new era of innovation and efficiency. By optimising production processes, improving product quality and promoting sustainability, AI offers a unique opportunity to make the steel industry fit for the future. Despite existing challenges, the potential for positive change is enormous. Close collaboration between industry, AI developers and educational institutions is key to successfully shaping this new era and realising both economic and environmental benefits.